In today’s data-driven world, businesses are constantly seeking ways to gain a competitive edge. One of the most powerful tools at their disposal is data warehousing. A well-designed data warehouse provides a centralized repository for all of your organization’s data, enabling you to access, analyze, and interpret information in ways that were previously impossible. But with so many different data warehousing solutions available, how do you choose the right one for your needs? This article will dive deep into the world of data warehousing, exploring the various solutions, their benefits, and how they can help you unlock valuable business insights.
From cloud-based data warehouses to on-premise solutions, the options are vast and complex. Understanding the key features, advantages, and limitations of each solution is crucial for making an informed decision. Whether you’re a small startup or a large enterprise, this guide will empower you to navigate the data warehousing landscape and choose the solution that best aligns with your business objectives and strategic goals. By leveraging the power of data warehousing, you can unlock a wealth of insights that will drive innovation, optimize operations, and ultimately lead to increased profitability.
Introduction: The Foundation of Data-Driven Decisions
In the contemporary business landscape, data reigns supreme. Organizations are increasingly realizing that harnessing the power of data is not just an advantage, but a necessity for survival and growth. This is where data warehousing solutions come into play.
Imagine having a centralized repository where all your critical business data resides, meticulously organized and readily available for analysis. This is the essence of data warehousing. By creating a single source of truth, organizations can gain invaluable insights into their operations, customer behavior, market trends, and more.
Data warehousing empowers businesses to make informed decisions based on concrete evidence, rather than gut feelings or anecdotal evidence. It provides a solid foundation for data-driven decision-making, leading to improved efficiency, enhanced customer experiences, and ultimately, greater profitability.
Understanding Data Warehousing: Concepts and Architecture
Data warehousing is a crucial aspect of modern business intelligence, enabling organizations to glean valuable insights from their data. A data warehouse is a central repository designed for storing, managing, and analyzing vast amounts of data from various sources, offering a comprehensive and holistic view of an organization’s operations.
Key Concepts of Data Warehousing
Understanding the fundamental concepts of data warehousing is essential for harnessing its power:
- Data Integration: Data from diverse sources, such as operational systems, transactional databases, and external data providers, is consolidated into a consistent format within the data warehouse.
- Data Transformation: Data undergoes cleaning, standardization, and transformation to ensure data quality and consistency.
- Data Modeling: The structure of the data warehouse is meticulously designed using multidimensional data models, facilitating efficient analysis and query processing.
- Data Analysis: Data warehouses empower users to perform complex queries, generate reports, and extract meaningful insights to guide strategic decision-making.
Data Warehouse Architecture
Data warehouses typically adhere to a layered architecture to facilitate effective data management and analysis. The core layers include:
Data Source Layer
This layer encompasses the diverse sources from which data is extracted, such as operational systems, transactional databases, and external data providers.
Extraction, Transformation, and Load (ETL) Layer
This layer handles the process of extracting data from sources, transforming it into the desired format, and loading it into the data warehouse.
Data Warehouse Layer
This layer houses the consolidated and transformed data, organized according to a predefined data model.
Data Mart Layer
Data marts are smaller, subject-oriented data repositories derived from the data warehouse, designed to cater to specific analytical needs.
Benefits of Data Warehousing
Organizations that leverage data warehousing reap numerous benefits, including:
- Improved Decision Making: Data warehousing provides a comprehensive view of business operations, enabling data-driven decision-making.
- Enhanced Business Insights: Organizations can identify trends, patterns, and anomalies in their data, unlocking valuable insights that drive business growth.
- Competitive Advantage: Data-driven insights enable organizations to respond rapidly to market changes and outpace competitors.
- Increased Efficiency: Streamlined data management and analysis processes optimize operational efficiency and resource allocation.
Data warehousing has revolutionized business intelligence, empowering organizations to harness the power of their data and gain a competitive edge. By understanding the fundamental concepts and architecture of data warehousing, organizations can effectively leverage this technology to drive informed decision-making and achieve strategic objectives.
Types of Data Warehousing Solutions
Data warehousing is a critical component of any organization’s data strategy, enabling businesses to gather, store, and analyze massive datasets to gain valuable insights. There are various data warehousing solutions available, each offering distinct advantages and catering to specific needs.
1. On-Premise Data Warehousing
On-premise data warehousing involves setting up and managing the entire data warehousing infrastructure within your organization’s physical premises. This approach provides complete control over your data and offers high levels of security and customization.
2. Cloud-Based Data Warehousing
Cloud-based data warehousing, also known as cloud data warehousing, leverages cloud computing services to store and analyze data. This option offers scalability, cost-effectiveness, and flexibility, as you can scale your resources up or down based on your needs. Major cloud providers, such as Amazon Web Services (AWS), Microsoft Azure, and Google Cloud Platform (GCP), offer comprehensive data warehousing solutions.
3. Hybrid Data Warehousing
Hybrid data warehousing combines the best of both on-premise and cloud-based solutions. This approach allows you to store and manage your critical data on-premise while leveraging cloud services for specific tasks, such as data analysis or backup. It offers a balance of control, security, and flexibility.
4. Data Lake
A data lake is a centralized repository for storing raw data in its native format. Unlike traditional data warehouses, data lakes don’t require upfront schema definitions, making them ideal for storing unstructured and semi-structured data. Data lakes are often used in conjunction with big data analytics tools and cloud storage services.
5. Data Mart
A data mart is a smaller, focused data warehouse that caters to the specific needs of a particular department or business unit. Data marts typically contain a subset of data from a larger data warehouse, making it easier to analyze and report on relevant information.
Choosing the right data warehousing solution depends on factors such as your organization’s size, data volume, budget, security requirements, and analytical needs. Each solution offers unique strengths and weaknesses, so careful consideration is essential to make an informed decision.
Benefits of Implementing a Data Warehouse
Data warehousing is a crucial aspect of modern business intelligence, offering a plethora of benefits that can significantly enhance decision-making and drive growth. By centralizing and organizing data from various sources, data warehouses provide a comprehensive view of business operations, enabling organizations to uncover valuable insights and make informed strategic choices.
One of the primary benefits of implementing a data warehouse is improved data quality. Data from disparate systems often varies in format, accuracy, and completeness. A data warehouse consolidates and standardizes data, ensuring consistency and reliability for analysis and reporting.
Data warehouses also foster enhanced decision-making by providing a holistic view of business performance. By analyzing historical and current data trends, organizations can identify patterns, predict future outcomes, and make strategic decisions based on data-driven insights.
Furthermore, a data warehouse enables better customer segmentation. By analyzing customer data, businesses can identify distinct customer groups based on their demographics, purchasing habits, and preferences. This segmentation allows for targeted marketing campaigns, personalized offers, and enhanced customer service.
Beyond these immediate benefits, data warehouses also contribute to increased efficiency and productivity. By automating data integration and analysis, organizations can streamline business processes, reduce manual effort, and free up valuable resources for strategic initiatives.
In conclusion, implementing a data warehouse can unlock a wealth of business insights, leading to improved decision-making, enhanced customer engagement, and increased efficiency. By embracing data warehousing, organizations can gain a competitive edge, drive growth, and achieve their business objectives.
Key Considerations for Choosing a Data Warehousing Solution
Data warehousing has become an essential component for businesses looking to gain valuable insights from their data. However, selecting the right data warehousing solution can be a complex process. To ensure a successful implementation, it’s crucial to consider several key factors.
Data Volume and Velocity: The amount and speed of data your business generates will significantly impact the solution you choose. Consider the volume of data you need to store and the velocity at which it’s generated. Some solutions are better suited for high-volume, real-time data processing, while others excel in handling large data sets with less frequent updates.
Scalability and Performance: As your business grows, your data warehousing needs will likely change. Choose a solution that can scale with your data growth and provide the necessary performance to meet your analytical requirements. Cloud-based solutions often offer greater scalability and flexibility.
Security and Compliance: Data security is paramount. Ensure the chosen solution adheres to relevant industry standards and regulations like HIPAA or GDPR. Look for features like data encryption, access control, and audit trails to protect sensitive information.
Integration and Compatibility: Seamless integration with existing systems and applications is crucial. The solution should be compatible with your current data sources, analytical tools, and reporting platforms.
Cost and Budget: Data warehousing solutions can range in cost from free open-source options to enterprise-grade platforms. Evaluate your budget and determine the cost-effectiveness of different solutions. Consider the total cost of ownership, including software licensing, hardware, maintenance, and support.
Ease of Use and Management: The solution should be user-friendly for both data analysts and business users. Consider the tools and features available for data manipulation, querying, and visualization. A well-designed user interface and intuitive management tools can simplify the process.
Vendor Support and Expertise: Choose a vendor with a proven track record and strong support services. Consider their experience, industry expertise, and the availability of training and documentation.
By carefully considering these factors, businesses can select a data warehousing solution that aligns with their specific needs and goals. The right solution will empower them to unlock valuable business insights, optimize operations, and drive informed decision-making.
Building a Robust Data Warehousing Strategy
In today’s data-driven world, organizations are increasingly relying on data warehousing solutions to gain insights and make informed decisions. However, building a robust data warehousing strategy requires careful planning and execution.
Here are some key considerations for building a robust data warehousing strategy:
- Clearly Define Business Goals and Requirements: The first step is to identify the specific business goals that the data warehouse will support. What insights do you need to achieve those goals? What data is required? Understanding these requirements will help you design a data warehouse that meets your needs.
- Choose the Right Data Warehouse Architecture: There are various data warehouse architectures available, each with its advantages and disadvantages. Consider factors like data volume, performance requirements, and scalability when choosing the right architecture. For example, a star schema is suitable for large data volumes and fast query processing, while a snowflake schema offers greater flexibility and scalability.
- Data Extraction, Transformation, and Loading (ETL): The ETL process is crucial for moving data from source systems into the data warehouse. Choose a reliable and efficient ETL tool that can handle the complexity of your data sources and transformations. Consider adopting a cloud-based ETL solution for scalability and flexibility.
- Data Quality and Governance: Data quality is paramount for accurate insights. Establish data governance policies and procedures to ensure data accuracy, consistency, and completeness. Implement data validation and cleansing processes to maintain data quality throughout the data warehousing lifecycle.
- Security and Compliance: Data security is of utmost importance in data warehousing. Implement strong access control measures and encryption to protect sensitive data. Ensure compliance with relevant regulations like GDPR and HIPAA.
- Monitoring and Performance Optimization: Continuously monitor data warehouse performance and identify bottlenecks. Optimize query performance, data loading, and storage to ensure efficient data processing and analysis. Utilize data warehousing tools for performance monitoring and optimization.
By following these key considerations, organizations can build a robust data warehousing strategy that enables them to unlock valuable business insights and drive informed decision-making. This strategic approach will help businesses gain a competitive edge in today’s data-driven landscape.
Data Integration and ETL Processes
Data integration is the process of combining data from multiple sources into a single, unified view. This is a crucial step in building a data warehouse, as it allows businesses to gain a holistic understanding of their data. The process typically involves cleaning, transforming, and loading data into a target system, often using Extract, Transform, and Load (ETL) tools.
ETL is a three-step process that involves:
- Extract: Extracting data from various source systems. These sources can include databases, spreadsheets, flat files, APIs, and other applications.
- Transform: Transforming the extracted data into a consistent format that aligns with the data warehouse’s schema. This might include data cleansing, data type conversions, and data aggregation.
- Load: Loading the transformed data into the data warehouse or data mart. This can be done in batch mode or real-time, depending on the business needs.
The efficiency and accuracy of the ETL process are critical to the success of any data warehousing initiative. If data is not properly integrated and transformed, it can lead to inaccurate insights and poor decision-making.
Here are some benefits of data integration and ETL processes:
- Improved Data Quality: ETL processes help ensure data consistency and accuracy, reducing errors and improving data quality.
- Enhanced Business Insights: Combining data from different sources allows for comprehensive analysis and deeper insights into business operations.
- Faster Decision-Making: Access to integrated and reliable data facilitates informed and timely decision-making.
- Increased Efficiency: Automation of ETL processes saves time and resources, enabling businesses to focus on higher-value activities.
Choosing the right ETL tools and techniques depends on factors such as data volume, complexity, and business requirements. There are a variety of ETL tools available, ranging from open-source solutions to enterprise-grade software.
In conclusion, data integration and ETL processes are essential for organizations looking to unlock the power of data warehousing. By effectively combining and transforming data, businesses can gain valuable insights, improve decision-making, and drive growth.
Data Modeling and Schema Design
In the realm of data warehousing, the foundation for extracting meaningful insights lies in effective data modeling and schema design. These processes are crucial for organizing, structuring, and storing vast amounts of data in a way that allows for efficient retrieval and analysis.
Data modeling is the process of creating a conceptual representation of the data, outlining its entities, attributes, and relationships. It essentially translates business requirements into a structured format, laying the groundwork for the actual database design.
Schema design, on the other hand, focuses on the technical implementation of the data model. It defines the table structures, data types, primary and foreign keys, and other database elements necessary to physically store and manage the data.
The success of a data warehousing solution hinges on the quality of data modeling and schema design. A well-designed schema ensures:
- Data integrity and consistency: Maintaining the accuracy and reliability of data by enforcing constraints and relationships.
- Efficient querying and analysis: Enabling quick and precise retrieval of information for analytical purposes.
- Scalability and flexibility: Accommodating growing data volumes and evolving business needs.
- Reduced redundancy and data duplication: Optimizing storage space and minimizing inconsistencies.
Several common data modeling methodologies, such as star schema and snowflake schema, are used in data warehousing. The choice of a particular methodology depends on factors like data complexity, performance requirements, and analytical needs.
In conclusion, data modeling and schema design are not merely technical exercises but crucial steps towards unlocking the true potential of data warehousing. A well-crafted data model and schema lay the foundation for extracting actionable insights and driving informed business decisions.
Data Quality Management and Governance
In the realm of data warehousing, the paramount importance of robust data quality management and governance cannot be overstated. These twin pillars form the bedrock for extracting meaningful insights and making informed business decisions.
Data quality refers to the accuracy, completeness, consistency, and timeliness of data. It ensures that information is reliable and trustworthy, enabling businesses to make accurate assessments and predictions. Data governance encompasses the policies, processes, and controls that ensure data is managed effectively throughout its lifecycle. This includes defining data ownership, establishing data security protocols, and implementing data standards.
Here’s how data quality management and governance contribute to unlocking business insights:
- Enhanced Decision Making: Accurate and reliable data empowers businesses to make informed decisions that drive growth and profitability. Poor data quality can lead to costly errors and missed opportunities.
- Improved Customer Experience: Data quality underpins personalized customer interactions. By leveraging accurate data, businesses can tailor products and services to individual needs, enhancing customer satisfaction.
- Increased Efficiency: Data governance streamlines data processes, reducing redundancies and improving efficiency. This translates into cost savings and faster time-to-market for new products and services.
- Enhanced Compliance: Data governance helps businesses comply with industry regulations and data privacy laws, mitigating legal and reputational risks.
- Competitive Advantage: Businesses with a robust data quality framework gain a competitive edge by leveraging insights that competitors may miss. They can identify emerging trends, anticipate customer needs, and innovate effectively.
In conclusion, data quality management and governance are essential components of a successful data warehousing strategy. By prioritizing data quality and establishing a strong governance framework, businesses can unlock valuable insights, make informed decisions, and achieve a competitive advantage in the data-driven world.
Data Security and Privacy in Data Warehousing
Data warehousing, a critical component of modern business intelligence, empowers organizations to harness the power of data for insightful decision-making. However, with the increasing volume and sensitivity of data stored in warehouses, ensuring data security and privacy becomes paramount. Striking a balance between accessibility and protection is vital for organizations to reap the benefits of data warehousing while safeguarding sensitive information.
Robust security measures are essential to prevent unauthorized access, data breaches, and data loss. Access control mechanisms, such as role-based access, limit user permissions to specific data sets, ensuring that only authorized individuals can view and manipulate sensitive information. Encryption, both at rest and in transit, safeguards data from malicious actors by converting it into an unreadable format. Regular security audits identify vulnerabilities and ensure compliance with industry standards, strengthening the overall security posture of the data warehouse.
Data privacy is another crucial aspect of data warehousing. Organizations must adhere to data privacy regulations such as the General Data Protection Regulation (GDPR) and the California Consumer Privacy Act (CCPA). Implementing data masking techniques obscures sensitive data elements while preserving data integrity and analytical value. Data anonymization removes identifiable information, protecting the privacy of individuals whose data is stored in the warehouse. Furthermore, adopting a privacy-by-design approach during data warehouse design and implementation ensures that privacy considerations are woven into the fabric of the solution.
In conclusion, data security and privacy are indispensable pillars of effective data warehousing. By prioritizing robust security measures, adhering to data privacy regulations, and adopting best practices, organizations can harness the power of data for strategic decision-making while protecting sensitive information and maintaining compliance.
The Future of Data Warehousing: Trends and Innovations
The landscape of data warehousing is rapidly evolving, driven by the exponential growth of data and the increasing demand for actionable insights. Organizations are embracing new technologies and methodologies to navigate this evolving landscape, leading to a future where data warehousing is more agile, efficient, and accessible than ever before.
One of the most significant trends is the rise of cloud-based data warehousing. Cloud platforms offer scalability, cost-effectiveness, and ease of deployment, making them an attractive option for businesses of all sizes. Cloud data warehouses also enable organizations to leverage advanced analytics and machine learning capabilities, further enhancing their ability to extract insights from their data.
Another key trend is the adoption of data lakes. Data lakes provide a centralized repository for all types of data, structured and unstructured, enabling organizations to store and analyze data from multiple sources in a single location. This approach fosters greater data exploration and discovery, leading to a more comprehensive understanding of business operations.
The increasing prominence of data governance is also shaping the future of data warehousing. Organizations are realizing the importance of establishing robust data governance frameworks to ensure data quality, security, and compliance. This includes defining data ownership, access controls, and data quality standards, ultimately driving trust and confidence in data-driven decision-making.
Furthermore, artificial intelligence (AI) and machine learning (ML) are playing an increasingly important role in data warehousing. AI and ML algorithms can automate data preparation, analysis, and reporting tasks, freeing up data analysts to focus on higher-value activities. These technologies can also identify patterns and anomalies in data, leading to more accurate predictions and insights.
The future of data warehousing promises to be a dynamic landscape characterized by innovation and evolution. By embracing these emerging trends and technologies, organizations can unlock new levels of business insights, optimize their operations, and drive competitive advantage in the data-driven era.
Data Warehouse Architecture
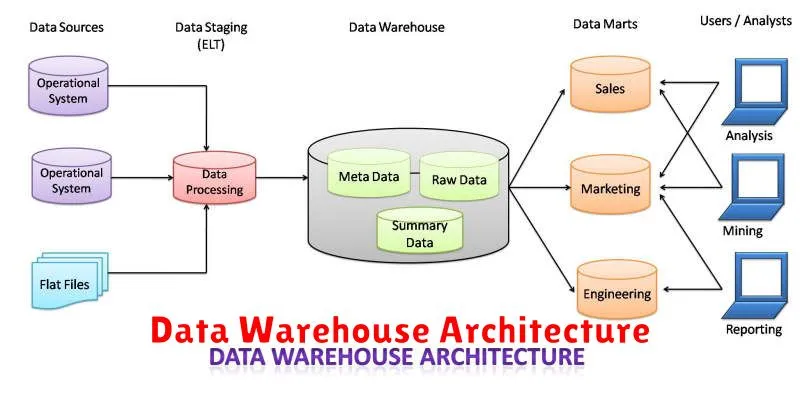
A data warehouse is a centralized repository of data from various sources, designed for analytical processing and reporting. Understanding the architecture of a data warehouse is crucial for effectively leveraging its potential. Here’s a breakdown of the key components:
Data Sources
Data warehouses gather data from diverse sources, including transactional databases, web logs, social media platforms, and external data providers. These sources are often heterogeneous, with varying data formats and structures.
Extraction, Transformation, and Loading (ETL)
The ETL process extracts data from source systems, transforms it into a standardized format, and loads it into the data warehouse. This crucial step ensures data consistency, quality, and readiness for analysis.
Data Warehouse Database
The heart of the data warehouse is the database, which stores the transformed data in a structured and organized manner. Common database management systems used in data warehouses include Oracle, SQL Server, and Teradata.
Metadata
Metadata provides essential information about the data stored in the warehouse, including data definitions, relationships, and quality attributes. It plays a critical role in understanding and interpreting the data.
Data Mart
Data marts are smaller, focused subsets of the data warehouse, tailored to specific business needs. They provide a more agile and efficient way to access and analyze data for particular departments or functions.
Reporting and Analytics Tools
Data warehouses are designed to support business intelligence and analytics. Various reporting and analytics tools can connect to the warehouse, providing users with interactive dashboards, visualizations, and advanced analytical capabilities.
Data Governance and Security
Data governance and security are essential to ensure the integrity, accuracy, and confidentiality of the data. Implementing access controls, data quality processes, and security measures is vital for maintaining data integrity and protecting sensitive information.
Types of Data Warehouses
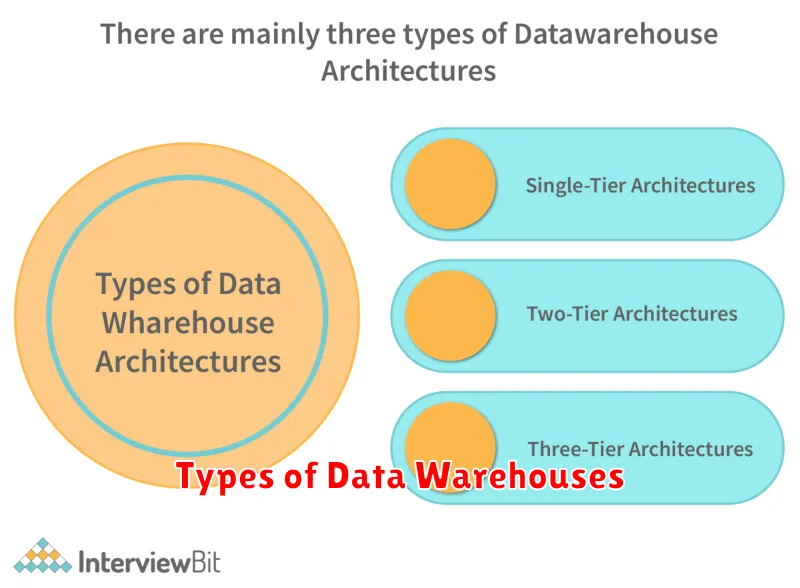
Data warehouses are crucial for businesses seeking to make sense of their vast data stores and gain valuable insights. These repositories gather data from various sources, cleanse it, and transform it into a structured format for analysis. But not all data warehouses are created equal. Understanding the different types of data warehouses available can help businesses choose the right solution for their specific needs.
Here’s a breakdown of common data warehouse types:
1. Enterprise Data Warehouse (EDW)
An EDW is a centralized repository that stores data from all business units within an organization. It’s designed to support complex reporting, analytics, and decision-making across the entire enterprise. EDWs are typically large-scale systems with high storage capacity and processing power, often using relational database management systems (RDBMS).
2. Data Mart
A data mart is a smaller, focused data warehouse that caters to the specific needs of a particular department or business function. It pulls data from the EDW or other sources, providing targeted insights for teams like marketing, sales, or finance. Data marts are often easier to implement and maintain compared to EDWs, making them a good option for departmental analysis.
3. Operational Data Store (ODS)
An ODS is a temporary storage area for operational data, typically used to support real-time decision-making and reporting. It’s designed for quick access and updates, enabling businesses to analyze recent data and make informed decisions based on the latest information. ODSs are often used in conjunction with EDWs, providing a layer of agility for operational analytics.
4. Data Lake
A data lake is a modern approach to data warehousing, storing raw data in its native format without any predefined schema. This allows for greater flexibility in analyzing diverse data types, including structured, semi-structured, and unstructured data. Data lakes are often used in conjunction with big data technologies, enabling businesses to explore and analyze massive datasets.
5. Cloud Data Warehouse
A cloud data warehouse is hosted on a cloud platform, offering scalability, elasticity, and cost-effectiveness. This type of data warehouse leverages the cloud’s infrastructure and resources to handle large volumes of data and complex analysis. Cloud data warehouses are increasingly popular, providing businesses with a flexible and efficient solution.
Choosing the right data warehouse type depends on factors such as the organization’s size, data volume, analytical needs, and budget. By carefully considering these factors, businesses can select the data warehousing solution that will unlock valuable insights and drive better decision-making.
Data Warehouse Benefits
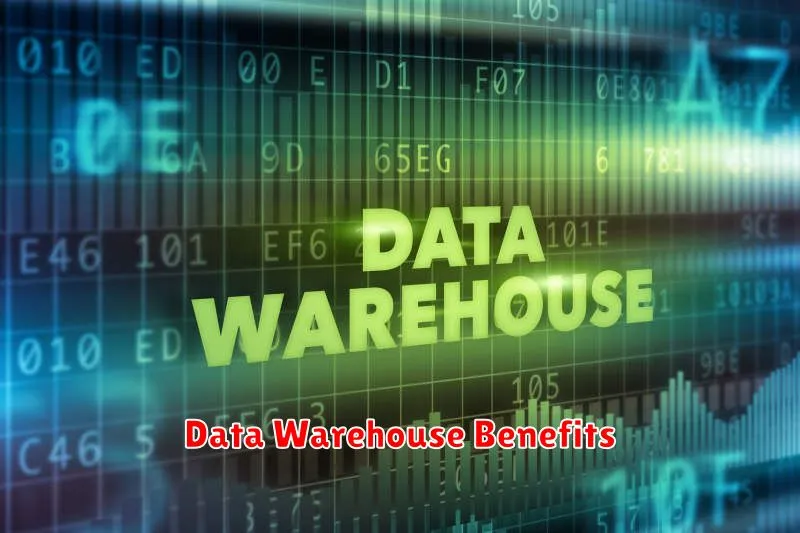
Data warehouses are powerful tools for businesses seeking to gain valuable insights from their data. By centralizing and integrating data from various sources, data warehouses provide a comprehensive view of an organization’s operations, enabling data-driven decision-making.
Here are some key benefits of implementing a data warehouse:
- Improved Decision Making: Data warehouses provide a unified platform for analyzing historical and current data, enabling businesses to identify trends, patterns, and anomalies that can inform strategic decisions.
- Enhanced Business Intelligence: By consolidating data from disparate systems, data warehouses facilitate the creation of insightful reports, dashboards, and visualizations that provide a holistic view of business performance.
- Increased Efficiency and Productivity: Data warehouses streamline data access and analysis, reducing the time and effort required to extract valuable information, leading to increased efficiency and productivity.
- Competitive Advantage: By leveraging data insights, businesses can gain a competitive advantage by identifying market opportunities, optimizing operations, and anticipating customer needs.
- Improved Customer Experience: Data warehouses enable businesses to understand customer behavior and preferences better, leading to personalized products and services that enhance customer satisfaction.
- Reduced Costs: Data warehouses can help businesses reduce costs by identifying areas for optimization, improving resource allocation, and reducing data redundancy.
In conclusion, data warehouses offer a myriad of benefits that can transform businesses. By harnessing the power of data, organizations can gain valuable insights, make informed decisions, and drive growth and innovation.
Cloud Data Warehouse
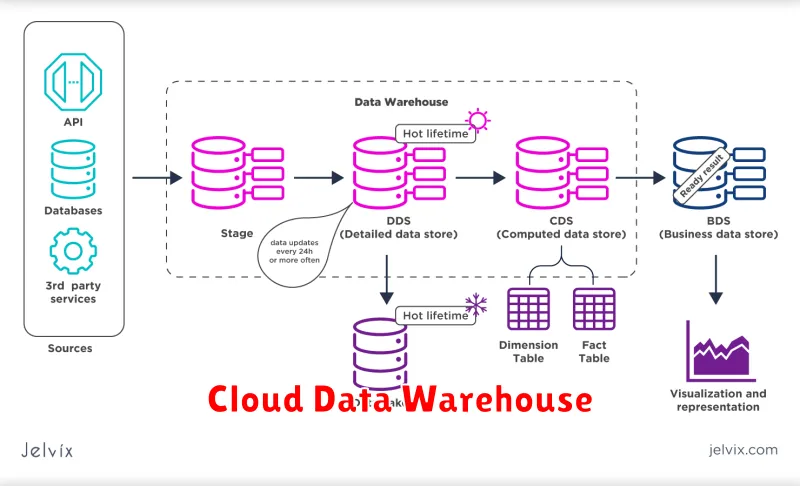
In today’s data-driven world, extracting meaningful insights from vast amounts of information is crucial for businesses to make informed decisions and gain a competitive edge. Cloud data warehouses have emerged as a powerful solution, offering a scalable, flexible, and cost-effective way to store, manage, and analyze data.
Traditional on-premises data warehouses often involve significant upfront investments in hardware and software, and scaling to meet increasing data demands can be challenging. Cloud data warehouses, on the other hand, provide a pay-as-you-go model, eliminating the need for large capital expenditures. They also offer automatic scaling capabilities, allowing businesses to adjust storage and compute resources as needed.
Key benefits of cloud data warehouses include:
- Scalability and Flexibility: Cloud data warehouses can easily scale up or down to accommodate changing data volumes and processing needs.
- Cost-Effectiveness: Pay-as-you-go pricing models eliminate upfront investments and reduce overall costs.
- Accessibility and Collaboration: Cloud-based solutions allow users to access data from anywhere with an internet connection, fostering collaboration among teams.
- Security and Reliability: Cloud providers offer robust security features and high availability, ensuring data protection and uptime.
- Integration with Analytics Tools: Cloud data warehouses seamlessly integrate with various analytics tools, enabling comprehensive data analysis.
Cloud data warehouses are revolutionizing how businesses manage and analyze their data, empowering them to unlock valuable insights and drive better decision-making. As data volumes continue to grow, cloud-based solutions will remain essential for businesses seeking to gain a competitive advantage in the digital age.
Data Warehouse Use Cases
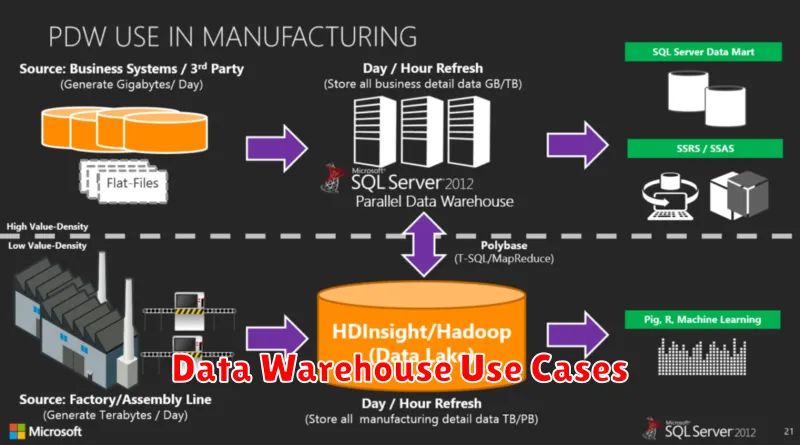
Data warehouses are a critical component of any organization’s data strategy, offering a centralized repository for storing and analyzing vast amounts of data. By integrating data from various sources, data warehouses empower businesses to gain valuable insights and make informed decisions. Here are some key use cases where data warehouses excel:
Customer Relationship Management (CRM):
Data warehouses enable businesses to understand customer behavior, preferences, and purchase history. This information can be leveraged to personalize marketing campaigns, enhance customer service, and develop targeted product recommendations. By analyzing customer data, businesses can improve customer retention and drive revenue growth.
Sales and Marketing Analytics:
Data warehouses provide a comprehensive view of sales performance, marketing campaign effectiveness, and customer acquisition costs. Businesses can analyze sales trends, identify high-performing campaigns, and optimize marketing strategies to maximize ROI. By understanding the impact of marketing initiatives, organizations can allocate resources effectively and achieve better outcomes.
Financial Analysis and Forecasting:
Data warehouses are invaluable for financial reporting, budgeting, and forecasting. By aggregating financial data from different departments and systems, businesses can gain a holistic view of their financial performance. This enables them to identify trends, predict future financial outcomes, and make informed decisions regarding investments, expenses, and risk management.
Operational Efficiency and Process Improvement:
Data warehouses can help businesses analyze operational data to identify bottlenecks, improve workflows, and enhance efficiency. By tracking key performance indicators (KPIs), organizations can gain insights into process performance and identify areas for optimization. This can lead to reduced costs, improved productivity, and enhanced customer satisfaction.
Competitive Intelligence:
Data warehouses allow businesses to monitor competitors, analyze market trends, and gain insights into industry dynamics. By leveraging external data sources and comparing their own performance to competitors, businesses can stay ahead of the curve and make strategic decisions.
In conclusion, data warehouses offer a wide range of use cases that empower businesses to unlock valuable insights and make data-driven decisions. By leveraging the power of data warehousing, organizations can gain a competitive advantage, optimize operations, and drive business growth.