In the ever-evolving landscape of business, making informed decisions based on real-time insights is crucial for achieving sustainable success. This is where predictive analytics comes into play. By leveraging the power of data, predictive analytics empowers businesses to anticipate future trends, identify potential risks, and optimize operations for maximum efficiency. This transformative approach enables companies to gain a competitive edge, make proactive decisions, and unlock new opportunities for growth.
From forecasting sales and demand to predicting customer churn and identifying fraud, predictive analytics has become an indispensable tool for businesses across various industries. By harnessing the power of data, companies can move beyond reactive decision-making and embrace a proactive approach that leverages insights to drive strategic initiatives. This article will delve deeper into the intricacies of predictive analytics, exploring its key applications, benefits, and the essential steps involved in implementing a successful strategy.
Understanding Predictive Analytics
In the realm of business, data is the new gold, and predictive analytics is the key to unlocking its immense value. It’s a powerful tool that allows businesses to analyze past and present data to predict future trends and outcomes. By harnessing the power of data, predictive analytics helps businesses make informed decisions, optimize operations, and ultimately achieve greater success.
At its core, predictive analytics involves employing sophisticated statistical techniques and algorithms to identify patterns and relationships within data. These insights are then used to develop models that can predict future events with a high degree of accuracy. The models are trained on historical data and continuously refined as new information becomes available, ensuring their ongoing relevance and predictive power.
The beauty of predictive analytics lies in its ability to go beyond simply describing the past. It empowers businesses to anticipate future possibilities, whether it’s forecasting sales, identifying customer churn, or predicting equipment failures. This proactive approach enables organizations to make timely adjustments, mitigate risks, and seize opportunities that might otherwise be missed.
Key Benefits for Businesses
Predictive analytics is a powerful tool that can help businesses of all sizes make better decisions, improve efficiency, and increase profitability. By analyzing historical data and identifying patterns, businesses can gain valuable insights into future trends and make informed decisions about everything from marketing campaigns to inventory management.
Here are some of the key benefits of using predictive analytics in business:
- Improved decision-making: Predictive analytics can help businesses make better decisions by providing them with a deeper understanding of their data and the ability to identify potential risks and opportunities.
- Increased efficiency: Predictive analytics can help businesses automate processes, optimize resources, and improve overall efficiency.
- Enhanced customer experience: Predictive analytics can help businesses understand their customers better and provide them with more personalized and relevant experiences.
- Reduced costs: Predictive analytics can help businesses reduce costs by identifying areas where they can improve efficiency and optimize their operations.
- Increased revenue: Predictive analytics can help businesses increase revenue by identifying new market opportunities and targeting their marketing campaigns more effectively.
- Improved risk management: Predictive analytics can help businesses identify potential risks and take steps to mitigate them.
- Enhanced competitiveness: Predictive analytics can help businesses stay ahead of the competition by identifying new trends and opportunities.
If you’re looking for a way to gain a competitive edge in today’s data-driven world, predictive analytics is a powerful tool that can help you achieve your business goals.
Common Use Cases
Predictive analytics finds its application across various business domains, empowering organizations to make informed decisions and optimize outcomes. Here are some common use cases:
Customer Relationship Management (CRM): Predictive analytics can identify customer churn risk, predict customer lifetime value, and personalize marketing campaigns to enhance customer engagement and loyalty.
Sales Forecasting: By analyzing historical data, predictive models can forecast future sales trends, optimize inventory levels, and allocate resources effectively.
Financial Risk Management: Predictive analytics plays a crucial role in identifying potential financial risks, detecting fraud, and optimizing credit scoring models.
Operations Optimization: Predictive analytics can improve operational efficiency by forecasting demand, optimizing supply chain logistics, and predicting equipment failures.
Healthcare: Predictive analytics can be used to predict patient readmission rates, identify high-risk patients, and personalize treatment plans.
Human Resources: Predictive analytics can help identify potential candidates for recruitment, predict employee turnover, and tailor training programs.
Marketing: Predictive analytics can optimize marketing campaigns by targeting the right audience, personalizing content, and predicting customer response.
Data Requirements and Preparation
Predictive analytics thrives on data, and the success of any model hinges on the quality and relevance of the data you feed it. The first crucial step is to identify the data requirements for your specific business problem. What variables are likely to influence the outcome you’re trying to predict? For instance, if you’re predicting customer churn, you might need data on customer demographics, purchase history, engagement levels, and customer support interactions.
Once you’ve identified the required data, it’s time for data preparation. This involves cleaning, transforming, and organizing the data to ensure it’s ready for analysis. This might include:
- Handling missing values: Impute missing data points or remove incomplete records.
- Data cleansing: Correct errors, inconsistencies, and duplicates.
- Data transformation: Standardize units, scale values, or create new features.
- Feature engineering: Create new features from existing data to improve model performance.
Remember, the cleaner and more relevant your data, the more accurate and insightful your predictive analytics models will be.
Choosing the Right Predictive Modeling Techniques
Predictive modeling is a powerful tool that can help businesses make better decisions and achieve greater success. By analyzing historical data, predictive models can identify patterns and trends that can be used to forecast future outcomes. However, choosing the right predictive modeling technique is crucial to ensure that the model is accurate, reliable, and effective.
The first step in choosing a predictive modeling technique is to clearly define the business problem you are trying to solve. What are you trying to predict? What factors are likely to influence the outcome? Once you have a clear understanding of the problem, you can start to consider different modeling techniques.
Some of the most common predictive modeling techniques include:
- Linear regression: This technique is used to predict a continuous outcome variable based on one or more predictor variables. It is a simple and widely used technique that can be effective for many problems.
- Logistic regression: This technique is used to predict a categorical outcome variable (e.g., yes/no, true/false) based on one or more predictor variables. It is often used in classification problems, such as predicting customer churn or fraud.
- Decision trees: This technique is used to create a tree-like structure that represents a series of decisions. Each node in the tree represents a decision point, and each branch represents a possible outcome. Decision trees are often used for classification problems, but can also be used for regression problems.
- Support vector machines (SVMs): This technique is used to find the optimal hyperplane that separates data points into different classes. SVMs are often used for classification problems, but can also be used for regression problems.
- Neural networks: This technique is inspired by the structure of the human brain. Neural networks are often used for complex problems, such as image recognition or natural language processing.
The best predictive modeling technique for a given problem will depend on a number of factors, including the type of data, the complexity of the problem, and the desired level of accuracy. It is important to consider all of these factors when choosing a modeling technique. In addition to the modeling technique itself, it is also important to consider the data preparation process. This includes tasks such as cleaning the data, handling missing values, and transforming variables. Proper data preparation is essential for building a robust and accurate predictive model.
Predictive modeling is a powerful tool that can help businesses make better decisions and achieve greater success. By carefully choosing the right modeling technique and preparing the data properly, businesses can unlock the potential of their data and gain valuable insights into the future.
Implementing Predictive Analytics Solutions
Predictive analytics is a powerful tool that can help businesses make better decisions, improve efficiency, and increase profitability. However, implementing predictive analytics solutions can be a complex undertaking. Here are some key steps involved in successfully deploying a predictive analytics solution:
1. Define your business problem: The first step is to clearly define the business problem you are trying to solve. What are your goals? What are the key metrics you want to improve? What data do you need to achieve these goals?
2. Gather and prepare your data: Once you have defined your business problem, you need to gather and prepare the data you need to solve it. This can be a challenging task, as data often comes from multiple sources and may need to be cleaned and formatted before it can be used.
3. Choose the right modeling techniques: There are many different predictive modeling techniques available, and the best choice will depend on your specific business problem and data. Some common techniques include linear regression, logistic regression, decision trees, and neural networks.
4. Build and evaluate your models: Once you have chosen your modeling techniques, you need to build and evaluate your models. This involves training the model on a set of historical data and then testing it on a separate set of data to see how well it predicts future outcomes.
5. Deploy and monitor your solution: Once your model has been validated, you can deploy it into production. This means integrating it into your existing systems and making it available to your users. It is also important to monitor your model over time to ensure that it continues to perform well and make adjustments as needed.
6. Communicate your findings: The results of your predictive analytics project should be communicated to stakeholders in a clear and concise way. This can include visualizations, reports, and presentations.
Implementing a predictive analytics solution is not a one-time event. It is an ongoing process that requires ongoing effort to maintain and improve your models. By following these steps, you can increase the likelihood of success with your predictive analytics initiatives.
Overcoming Challenges and Pitfalls
Predictive analytics holds immense potential for businesses, but its implementation isn’t without its challenges. Successfully navigating these hurdles is crucial for reaping the full benefits of data-driven insights.
One of the primary obstacles is data quality. Incomplete, inaccurate, or inconsistent data can lead to flawed predictions and undermine the entire process. Addressing this requires establishing robust data management practices, including data cleansing, validation, and integration.
Another significant challenge is model complexity. Building predictive models often involves intricate algorithms that require specialized expertise. Selecting the right model and tuning its parameters to optimize performance can be a complex task, demanding collaboration between data scientists and business stakeholders.
Furthermore, interpretation bias can creep into the analysis. Human biases may unconsciously influence the selection of variables or the interpretation of results, leading to inaccurate predictions. Establishing clear guidelines and employing rigorous validation techniques can help mitigate this risk.
Finally, organizational resistance to change can hinder the adoption of predictive analytics. Skepticism about the value of data-driven insights and concerns about job displacement can create resistance. Addressing these concerns through clear communication, training, and demonstrating tangible benefits is essential.
By proactively addressing these challenges and pitfalls, businesses can unlock the full potential of predictive analytics and harness the power of data to drive future success.
Measuring Success and ROI
Measuring the success and return on investment (ROI) of predictive analytics is crucial for demonstrating its value and securing ongoing support within a business. A comprehensive approach to evaluating its effectiveness involves considering multiple key metrics:
- Accuracy and Precision: Assessing the accuracy of predictions is essential. This involves evaluating how closely the predicted outcomes align with actual results. A high level of accuracy indicates the model’s ability to reliably anticipate future trends and behaviors.
- Business Impact: Quantifying the tangible benefits of using predictive analytics is essential. This could involve measuring improvements in key performance indicators (KPIs) such as sales, customer satisfaction, or operational efficiency.
- Cost Savings: Identifying cost reductions achieved through predictive analytics is vital. This could include reduced inventory levels, optimized staffing, or minimized risk of fraud.
- Return on Investment (ROI): Calculating the ROI of predictive analytics involves comparing the total cost of implementing and maintaining the system with the financial gains generated by its insights.
By carefully tracking these metrics, businesses can gain a clear understanding of the true value and impact of their predictive analytics initiatives. This data-driven approach enables them to justify investments, optimize model performance, and maximize the return on their data analytics efforts.
Real-World Examples of Predictive Analytics in Action
Predictive analytics is transforming how businesses operate, offering insights that were once unimaginable. By leveraging historical data and powerful algorithms, organizations can forecast future trends, optimize operations, and make data-driven decisions that drive growth and efficiency.
Here are some compelling real-world examples of how predictive analytics is making a tangible impact across various industries:
Retail
Personalized Recommendations: Online retailers like Amazon and Netflix use predictive analytics to recommend products and content tailored to individual customer preferences. By analyzing browsing history, purchase patterns, and other data points, they can offer highly relevant suggestions that increase customer engagement and drive sales.
Healthcare
Disease Prediction: Predictive models are being employed to identify patients at risk of developing certain diseases, enabling early intervention and preventive care. By analyzing medical records, lifestyle factors, and genetic data, healthcare providers can proactively address potential health issues before they become serious.
Finance
Fraud Detection: Banks and financial institutions use predictive models to detect fraudulent transactions in real-time. By analyzing patterns in transaction data, they can identify suspicious activities and take immediate action to prevent financial losses.
Manufacturing
Predictive Maintenance: Predictive analytics plays a crucial role in optimizing equipment maintenance schedules. By analyzing sensor data from machines, manufacturers can anticipate potential failures and schedule maintenance proactively, minimizing downtime and maximizing production efficiency.
Marketing
Targeted Advertising: Predictive models enable marketers to create highly targeted advertising campaigns. By analyzing demographics, interests, and online behavior, they can deliver personalized messages that resonate with specific audience segments, resulting in higher conversion rates.
These are just a few examples of the transformative power of predictive analytics. As data continues to grow exponentially, the applications of this technology will only expand, unlocking new possibilities and driving innovation across industries.
Future Trends in Business Forecasting
Predictive analytics is revolutionizing business forecasting, allowing companies to make data-driven decisions and gain a competitive edge. As technology advances and data becomes more readily available, the future of business forecasting is bright, with several exciting trends emerging.
Artificial Intelligence (AI) and Machine Learning (ML) will play a more prominent role in forecasting. AI algorithms can analyze vast amounts of data, identify patterns, and generate accurate predictions. This will enable businesses to automate forecasting tasks, improve accuracy, and gain valuable insights that would otherwise be missed.
Real-time forecasting will become increasingly important as businesses strive for agility and responsiveness. With real-time data streams, companies can monitor changes in market conditions and adjust their forecasts accordingly, allowing for quicker decision-making and improved adaptability.
Scenario planning will gain prominence as a powerful tool for forecasting under uncertainty. This involves creating and analyzing multiple scenarios to assess potential outcomes and develop contingency plans. This approach helps businesses navigate complex and volatile environments and make informed decisions in the face of uncertainty.
Cloud-based forecasting solutions will continue to grow in popularity. These solutions offer scalability, flexibility, and affordability, making them ideal for businesses of all sizes. Cloud-based platforms also provide access to advanced analytics tools and real-time data feeds, enhancing forecasting capabilities.
Integration with other business systems will be crucial for maximizing the value of predictive analytics. Connecting forecasting models to CRM, ERP, and other systems will allow for a more holistic view of business operations and facilitate data-driven decision-making across all departments.
The future of business forecasting holds exciting possibilities. By embracing these trends, businesses can unlock the full potential of predictive analytics and gain a competitive edge in an increasingly data-driven world.
Ethical Considerations in Predictive Analytics
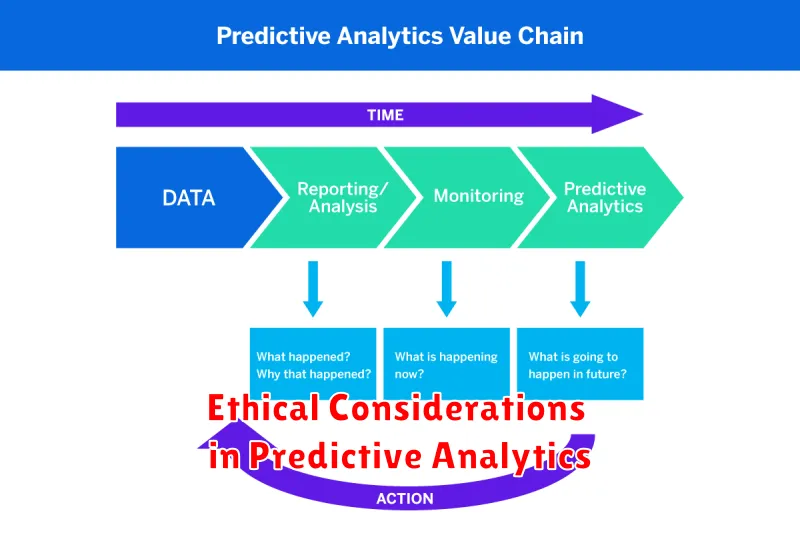
Predictive analytics is a powerful tool that can be used to gain valuable insights from data and make informed decisions about the future. However, as with any powerful tool, it’s important to use predictive analytics ethically. Failure to do so can lead to negative consequences for individuals, organizations, and society as a whole.
One of the most important ethical considerations in predictive analytics is bias. Data used to train predictive models can often reflect existing societal biases, which can then be perpetuated and amplified by the models themselves. For example, a model used to predict loan applications might be biased against certain demographics if the training data reflects historical lending practices that were discriminatory.
Another important ethical consideration is privacy. Predictive analytics often involves collecting and analyzing large amounts of personal data. It’s crucial to ensure that this data is collected and used responsibly and ethically. This includes obtaining informed consent from individuals, protecting their data from unauthorized access, and being transparent about how their data is being used.
It’s also important to consider the transparency and explainability of predictive models. Users should be able to understand how the models work and what factors are driving their predictions. This is especially important in situations where the models are being used to make high-stakes decisions, such as in healthcare or criminal justice.
Finally, it’s important to consider the potential for misuse of predictive analytics. For example, a model that predicts customer churn could be used to unfairly target certain groups of customers with aggressive marketing tactics. It’s important to ensure that predictive analytics is used for good and not for harm.
By carefully considering these ethical considerations, we can help ensure that predictive analytics is used responsibly and effectively to benefit individuals, organizations, and society as a whole.
The Role of Data Scientists and Analysts
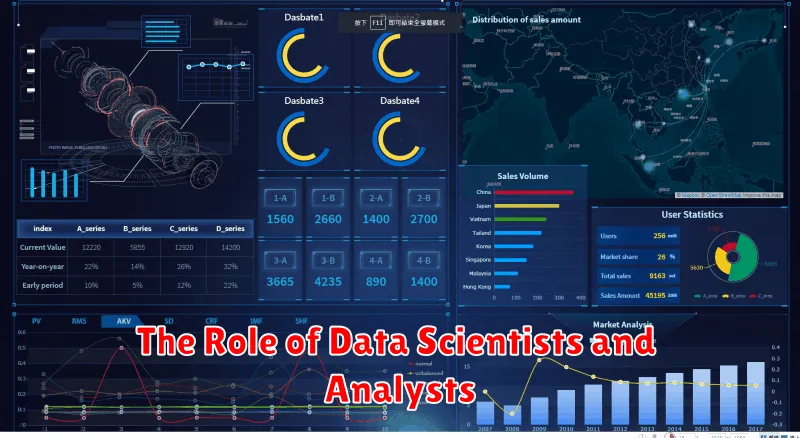
In the realm of predictive analytics, data scientists and data analysts play crucial roles in extracting valuable insights from data and transforming them into actionable strategies. Data scientists, armed with their expertise in advanced statistical techniques, machine learning algorithms, and data visualization, delve deep into complex data sets to uncover hidden patterns and predict future trends. They are the architects of predictive models, building sophisticated algorithms that can forecast customer behavior, market fluctuations, and operational efficiencies.
Data analysts, on the other hand, focus on interpreting and presenting data in a clear and concise manner. They use their analytical skills to transform raw data into meaningful insights, identifying key performance indicators and presenting them in dashboards and reports. Data analysts are the storytellers of data, bridging the gap between complex technical information and actionable business strategies.
Together, data scientists and analysts form a powerful duo that empowers businesses to make informed decisions based on data-driven insights. Their collaborative efforts ensure that data is not just collected but also harnessed to drive future success.
Integrating Predictive Analytics with Business Intelligence
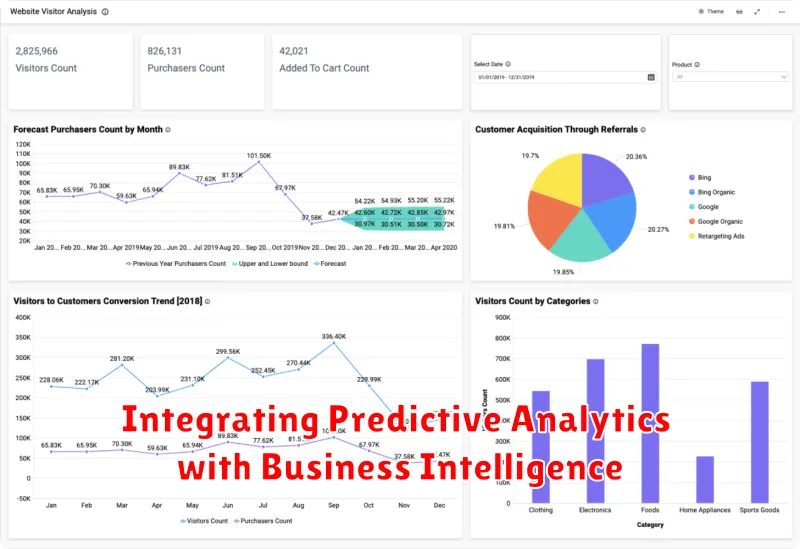
In the era of big data, businesses are increasingly recognizing the value of predictive analytics in driving informed decisions. Predictive analytics leverages historical data and statistical techniques to forecast future outcomes and identify potential trends. However, maximizing the impact of predictive analytics requires seamless integration with business intelligence (BI) platforms.
Business Intelligence provides a comprehensive view of current business operations, enabling data visualization, reporting, and analysis. Integrating predictive analytics into BI tools allows organizations to unlock the full potential of their data by combining historical insights with future predictions. This integration empowers businesses to:
- Proactive decision-making: Predictive models can identify potential risks and opportunities before they materialize, enabling businesses to take proactive steps to mitigate risks or capitalize on emerging trends.
- Improved forecasting accuracy: By incorporating predictive analytics, BI systems can generate more accurate forecasts for sales, demand, inventory levels, and other key metrics, leading to better resource allocation and operational efficiency.
- Enhanced customer segmentation: Predictive models can segment customers based on their purchasing behavior, preferences, and lifetime value, allowing businesses to tailor marketing campaigns and provide personalized experiences.
- Automated insights: Integrating predictive analytics into BI dashboards can automate the process of identifying key insights and patterns, freeing up analysts to focus on strategic decision-making.
Integrating predictive analytics into BI involves several key considerations:
- Data integration: Ensuring that historical data from BI systems is accessible for predictive modeling is crucial. This may require data cleaning, transformation, and standardization.
- Model selection: Choosing appropriate predictive models based on the specific business problem and available data is essential. Different models have varying strengths and weaknesses.
- Visualization and reporting: Presenting predictive insights in a clear and actionable manner is critical. BI tools should be able to visualize model outputs and generate reports that are easily understood by decision-makers.
- Collaboration and communication: Effective integration requires close collaboration between data scientists, BI analysts, and business stakeholders to ensure alignment on goals and interpretation of results.
By seamlessly integrating predictive analytics with business intelligence, organizations can leverage the power of their data to make smarter decisions, optimize operations, and gain a competitive advantage. This integration empowers businesses to move beyond reactive decision-making and embrace a future-oriented approach driven by data-driven insights.
Top Predictive Analytics Tools for Business
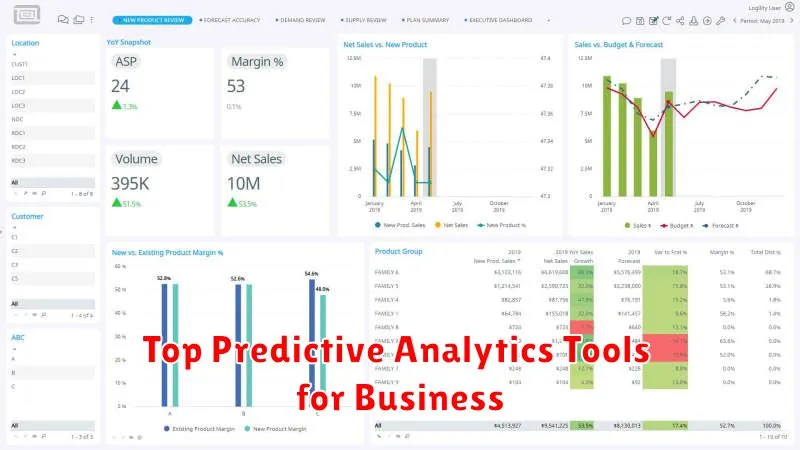
Predictive analytics has become an essential tool for businesses looking to gain a competitive edge. By analyzing historical data and identifying patterns, these tools can help predict future trends and outcomes, allowing companies to make informed decisions and optimize their operations.
Here are some of the top predictive analytics tools for business:
1. Microsoft Azure Machine Learning
Azure Machine Learning is a cloud-based platform that provides a comprehensive suite of tools for building, deploying, and managing predictive models. It offers a wide range of algorithms, pre-built models, and integration with other Azure services, making it a powerful option for businesses of all sizes.
2. Amazon Machine Learning
Amazon Machine Learning is another cloud-based platform that simplifies the process of building and deploying predictive models. It provides user-friendly interfaces and pre-built models, making it accessible even for users without extensive machine learning expertise.
3. Google Cloud AI Platform
Google Cloud AI Platform is a comprehensive machine learning service that offers a wide range of tools and features for building and deploying predictive models. It leverages Google’s advanced AI capabilities, providing high-performance and scalable solutions.
4. IBM Watson Studio
IBM Watson Studio is a data science and machine learning platform that provides a collaborative environment for building and deploying predictive models. It offers a wide range of tools and features, including data visualization, model building, and deployment.
5. RapidMiner
RapidMiner is a comprehensive data science platform that provides a user-friendly interface for building and deploying predictive models. It offers a wide range of algorithms, pre-built models, and automation features, making it a popular choice for both data scientists and business users.
6. Alteryx
Alteryx is a data analytics platform that provides a drag-and-drop interface for building and deploying predictive models. It offers a wide range of pre-built tools and connectors, making it easy to integrate with various data sources and systems.
Choosing the right predictive analytics tool depends on your specific needs and requirements. Consider factors such as the complexity of your data, your budget, and your team’s technical expertise.
Getting Started with Predictive Analytics: A Step-by-Step Guide
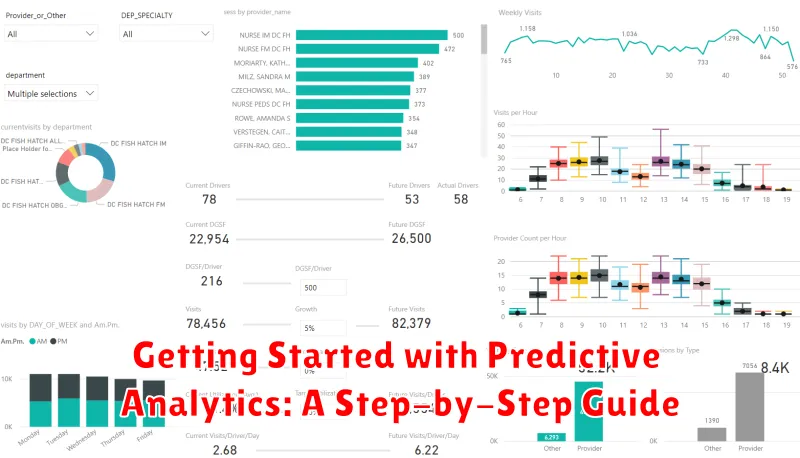
Predictive analytics is a powerful tool that can help businesses make better decisions by using data to predict future outcomes. But where do you start? This step-by-step guide will walk you through the basics of getting started with predictive analytics.
1. Identify your business goals. What problems are you trying to solve? What questions do you need answers to? Once you have a clear understanding of your goals, you can start to identify the data that will be most helpful in achieving them.
2. Gather and prepare your data. This is a crucial step in the process. Your data needs to be accurate, relevant, and complete. You may need to clean and transform your data before you can use it for predictive analytics.
3. Choose a predictive model. There are many different types of predictive models, so it’s important to choose the one that’s best suited for your needs. Consider factors such as the type of data you have, the complexity of the problem, and the level of accuracy you require.
4. Train and evaluate your model. Once you’ve chosen a model, you need to train it on your data. This involves feeding the model your data and allowing it to learn patterns and relationships. Once the model is trained, you need to evaluate its performance to ensure it’s accurate.
5. Deploy and monitor your model. Once you’re satisfied with the performance of your model, you can deploy it and start using it to make predictions. It’s important to monitor the model’s performance over time and make adjustments as needed.
Getting started with predictive analytics may seem daunting, but by following these steps, you can start harnessing the power of data to make better decisions and achieve your business goals.